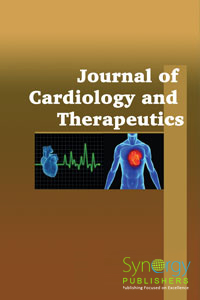
Implementation of a Computer-Based Decision Support System for Outcomes Prediction and Clinical Triage: Initial Results of Two Pilot Studies Pages 71-77
Hisham M.F. Sherif, Sharmila S. Johnson and Stephanie A. Klair
Department of Cardiac Surgery, Christiana Hospital, Christiana Care Health System, Newark, Delaware, USA
DOI: http://dx.doi.org/10.12970/2311-052X.2013.01.02.6
Download PDFAbstract: Objective: To develop a custom-built, computer-based clinical decision-support tool to help determine the underlying cause of the patient’s clinical presentation, its severity level and predicted outcome.
Materials and Methods: A novel, customized, computer-based decision-support tool, rooted in the Bayesian Network technology was constructed. Based on documented data from patients’ medical records, the probabilistic predictive model was utilized to validate patients’ triage in two different clinical scenarios:
1. Prediction of neurologic deficits in patients resuscitated from cardiac arrest following an Acute Coronary Syndrome/ST-Elevation Myocardial Infarction (ACS/STEMI), and thus likelihood of benefit from (or futility of) emergent coronary revascularization. Study group consisted of 99 patients resuscitated from NSTEMI. For this particular study, the endpoints were in-hospital death, neurologic recovery and the accurate prediction of outcomes by the program.
2. Prediction of the likelihood of presence of and readmission for Acute Decompensated Heart Failure syndrome. Study groups consisted of 20 randomly generated patient clinical profiles (Phase I) and 100 cases from our emergency room electronic medical records: 55 cases of acute heart failure, 14 cases of pneumonia, 7 cases of COPD exacerbation and 11 cases of “other” conditions. End points were the accurate prediction of the underlying diagnosis and the correct disposition.
In both studies, observed data from our institutional electronic medical records registry was utilized to populate the computer program and to validate its initial results. Subsequently, multiple, random clinical case scenarios were used to validate the predictive properties of the computer tool (Phase I) Finally (Phase II), the program was tested using real-world data from our institutional emergency room electronic medical records.
Results: First study: Overall, 64 (65%) patients survived, while 35 (35%) died. On initial examination, 25% of patients were alert, 14% were minimally responsive and 60% were unresponsive.
First Group (5 Hypothetical Case scenarios): Diagnostic accuracy rate 4/5 (80%)
Study Cohort (99 cases) 95/99% (95%); Overall Accuracy: 87%
Second study:
Phase I: Diagnostic accuracy rate of 85%; Disposition accuracy rate of 80%; Overall Accuracy rate 82%
Phase II: Diagnostic accuracy rate of 98%; Disposition accuracy rate of 91%; Overall accuracy rate of 94%
Conclusions: A custom-built, computer based predictive model, utilizing a probability engine software and based on population-wide real-life clinical data, can be a useful adjunct in clinical decision making and medical triage. This takes special significance in such high-risk, fast-paced environments such as the emergency department and the intensive care unit.
Keywords: Predictive modeling, Bioinformatics, Clinical Risk Assessment, Clinical Decision-making. Read more